Wed, 16 Apr 2025
–
16:00
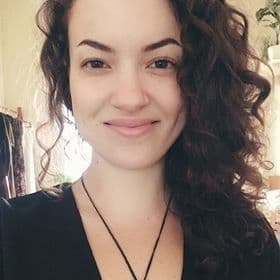
University of Pittsburgh
Facial expressions are inherently dynamic, and our visual system is sensitive to subtle changes in their temporal sequence. However, researchers often use dynamic morphs of photographs—simplified, linear representations of motion—to study the neural correlates of dynamic face perception. To explore the brain's sensitivity to natural facial motion, we constructed a novel dynamic face database using generative neural networks, trained on a verified set of video-recorded emotional expressions. The resulting deepfakes, consciously indistinguishable from videos, enabled us to separate biological motion from photorealistic form. Results showed that conventional dynamic morphs elicit distinct responses in the brain compared to videos and photos, suggesting they violate expectations (n400) and have reduced social salience (late positive potential). This suggests that dynamic morphs misrepresent facial dynamism, resulting in misleading insights about the neural and behavioural correlates of face perception. Deepfakes and videos elicited largely similar neural responses, suggesting they could be used as a proxy for real faces in vision research, where video recordings cannot be experimentally manipulated. And yet, despite being consciously undetectable as fake, deepfakes elicited an expectation violation response in the brain. This points to a neural sensitivity to naturalistic facial motion, beyond conscious awareness. Despite some differences in neural responses, the realism and manipulability of deepfakes make them a valuable asset for research where videos are unfeasible. Using these stimuli, we proposed a novel marker for the conscious perception of naturalistic facial motion – Frontal delta activity – which was elevated for videos and deepfakes, but not for photos or dynamic morphs.